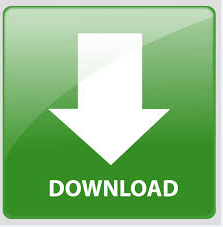
Variations in the relation between education and cause-specific mortality in 19 European populations: a test of the ‘fundamental causes’ theory of social inequalities in health. Education and cause-specific mortality: the mediating role of differential exposure and vulnerability to behavioral risk factors. Education, Health and Mortality: Evidence from a Social Experiment (National Bureau of Economic Research, 2012). Education and adult cause-specific mortality-examining the impact of family factors shared by 871 367 Norwegian siblings. LD score regression distinguishes confounding from polygenicity in genome-wide association studies. Estimates of the economic return to schooling for the United Kingdom. Comparison of sociodemographic and health-related characteristics of UK Biobank participants with those of the general population. The causal effect of education on wages revisited: the causal effect of education. Education and Health: Evaluating Theories and Evidence (National Bureau of Economic Research, 2006).ĭickson, M. The effect of education on adult mortality and health: evidence from Britain. Causal effect of education on mortality in a quasi-experiment on 1.2 million Swedes. We found consistent evidence that remaining in school causally reduced the risk of diabetes and mortality in all specifications. We used a regression discontinuity design to investigate the causal effects of remaining in school. We exploit the raising of the minimum school leaving age in the UK in September 1972 as a natural experiment 6. Here, we add to the evidence using data from a large sample from the UK Biobank 5. Previous studies have exploited this natural experiment using population-level administrative data to investigate mortality, and surveys to investigate the effect on morbidity 1, 2, 4. Natural experiments, such as laws that increase the minimum school leaving age, are a potentially more robust source of evidence about the causal effects of education. Much of the evidence about the effects of education comes from observational studies, which can be affected by residual confounding. Problems computing the test.Educated people are generally healthier, have fewer comorbidities and live longer than people with less education 1, 2, 3. "Note: the rank of the differenced variance matrix (0) does not equal the number of coefficients being tested (9) be sure this is what you expect, or there may be The coefficients of Random Model is the same of Fixed Model and there is a message saying: I'm facing some problems with Hausman's Test. sigmamore or sigmaless are recommended when comparing fixed-effects and random-effects linear regression because they are much less likely to produce a non–positive-definite-differenced covariance matrix (although the tests are asymptotically equivalent whether or not one of the options is specified).
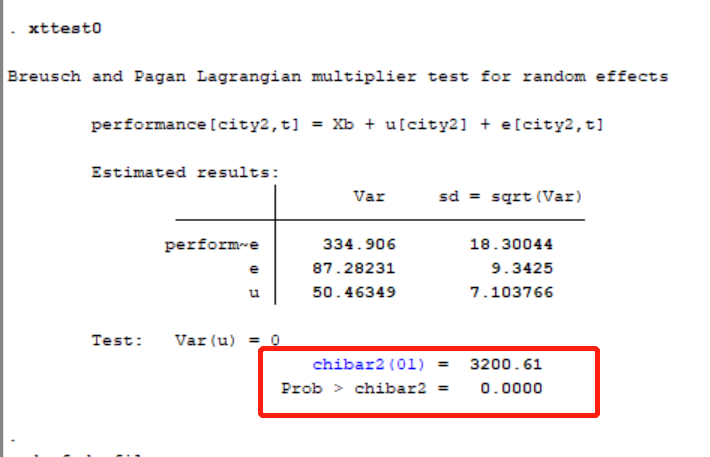
e(rmse) is stored after the xtreg command with the re option. e(sigma e) is stored after the xtreg command with the fe or mle option. These options can be specified only when both estimators store e(sigma) or e(rmse), or with the xtreg command. sigmaless specifies that the covariance matrices be based on the estimated disturbance variance from the consistent estimator. This option provides a proper estimate of the contrast variance for so-called tests of exogeneity and overidentification in instrumental-variables regression.
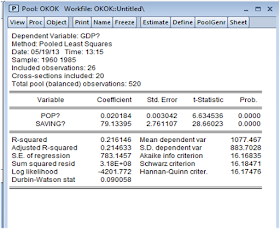
sigmamore specifies that the covariance matrices be based on the estimated disturbance variance from the efficient estimator. Sigmamore and sigmaless specify that the two covariance matrices used in the test be based on a common estimate of disturbance variance (σ 2 ). Many thanks for your help, I really appreciate it! I noticed online that squared variables could be centred to avoid multicollinearity but also found a lot of argument against this. I created the experience squared variable by typing the commands gen experiencesquared=experience^2. I'm sorry I'm not too familiar with all the terminology as this is the first time I am doing my own regressions so I'm not sure what you mean. I didn't try suest as I saw online this isn't for panel data. Is this just by chance they are insignificant in the fixed effects model, or does it have something to do with the Hausman test not working? I did add sigmamore and did get the result that I had to use fixed effects.īut, I was wondering, what are the implications of using sigmamore? If I write about in my project, would I say I used sigmamore and would I need to justify why, or would I just say that the Hausman test showed I needed to use fixed effects so I did?Īlso, after using fixed effects, some variables become almost completely insignificant.

You may want to add -sigmamore- option to -hausman- or try -suest-.Īs an aside: have you created squared terms via -fvvarlist- or by hand?Thanks a lot for your response Carlo.
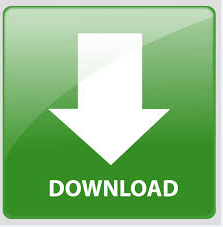